Students' accounts of published articles
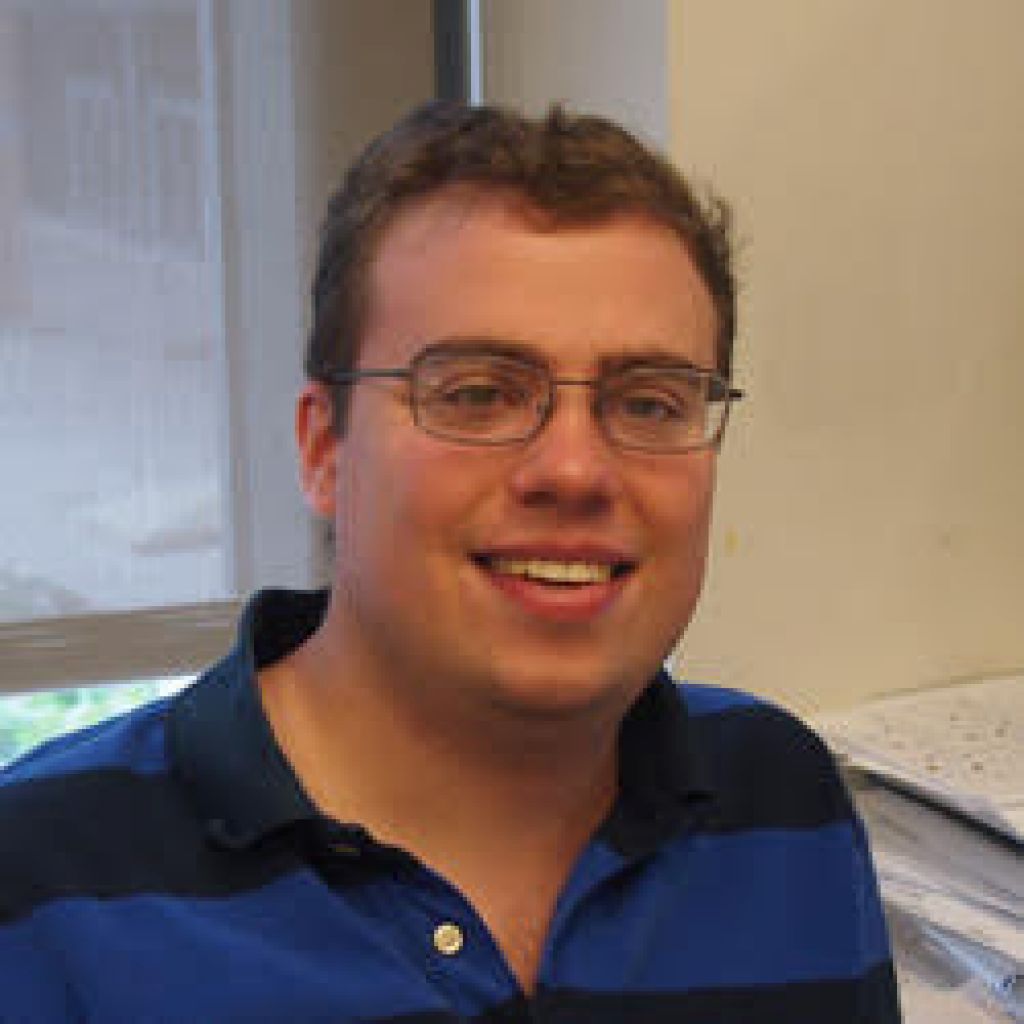
Complex genetic interactions account for developmental defects of the 16p11.2 deletion
Summary by Matthew Jensen: Copy-number variations (CNVs), or large deletions and duplications in the genome which span multiple genes, have been associated with numerous neurodevelopmental disorders, including autism, schizophrenia, and intellectual disability. Identifying causative genes within syndromic CNVs with invariable phenotypes typically involves mapping critical regions in individuals with atypical CNV breakpoints and then characterizing candidate genes in animal models. However, many CNVs contribute to a wide range of variable phenotypes and may even be found in mildly-affected or unaffected individuals. For example, the 16p11.2 deletion is responsible for 1% of all autism cases, yet only 40% of individuals with the deletion have an autism phenotype. This suggests that these variably-expressive CNVs do not have a single causative gene, but that multiple genes may have a combinatorial effect on neurodevelopment.
To screen for neurodevelopmental defects among 16p11.2 genes, we used RNA interference (RNAi) to knock down expression of conserved 16p11.2 homologs in Drosophila melanogaster. The use of fruit fly models proved valuable for the large-scale genetic screen, as the study entailed 565 individual and pairwise knockdown experiments, far more than the number of screens that could be efficiently performed in mice. An initial screen of ubiquitous and tissue-specific knockdowns found that most tested 16p11.2 homologs led to neuronal or developmental defects. We next used Flynotyper, a software previously developed by BG student Qingyu Wang, to quantify rough eye phenotypes in eye-specific knockdowns of 16p11.2 homologs. Knockdown of most homologs led to rough eye phenotypes, which we found were attributed to defects in cell proliferation early in larval development leading to abnormal cell counts and organization in the adult eye. We then screened for interactions between 16p11.2 homologs using Flynotyper, and found 24 pairwise interactions that either enhanced or suppressed the proliferation defects seen in the one-hit knockdowns. A further 46 interactions were identified between 16p11.2 homologs and homologs of known neurodevelopmental genes or genes in other variably-expressive CNV regions. We also analyzed RNA-sequencing data from six 16p11.2 homologs and validated 18 predicted interactions with differentially-expressed genes using knockdown flies. Finally, we mapped the 16p11.2 homologs and 35 interacting genes to a human brain-specific gene interaction network and found an enrichment for cell proliferation function among the connecting neighbor genes.
Overall, we identified 24 additive or epistatic interactions among 16p11.2 genes and 64 interactions between 16p11.2 genes and important neurodevelopmental genes, suggesting a pervasive interaction-based model for pathogenicity of the deletion. These interactions occur through shared cell proliferation pathways, suggesting that variants outside the CNV in these pathways are responsible for the wide variability of features in individuals with the deletion. Drugs targeting cell proliferation pathways could therefore be tested for treatment of this CNV. Finally, this study illustrates the importance of large-scale functional screening of genetic interactions in the developing nervous system, which would be useful for identifying genetic interactions in other variably-expressive CNVs.
This study was recently published in Nature Communications. The project was led by BMB post-docs Janani Iyer and Dhruba Singh, BG graduate student Matthew Jensen, and Biology undergraduate student Payal Patel, all advised by Santhosh Girirajan. Other collaborators included Melissa Rolls at Penn State, Arjun Krishnan at Michigan State University, John Manak at the University of Iowa, and Jose Badano at Institut Pasteur de Uruguay. The work was supported by the Computation, Bioinformatics, and Statistics (CBIOS) training grant at Penn State, the National Institutes of Health, the March of Dimes Foundation, the Brain and Behavior Research Foundation, and the Huck Institutes of the Life Sciences at Penn State.
Iyer J, Singh MD, Jensen M, Patel P, Pizzo L, Huber E, Koerselman H, Weiner AT, Lepanto P, Vadodaria K, Kubina A, Wang Q, Talbert A, Yennawar S, Badano J, Manak JR, Rolls MM, Krishnan A, Girirajan S. Pervasive genetic interactions modulate neurodevelopmental defects of the autism-associated 16p11.2 deletion in Drosophila melanogaster. Nat Commun. 2018 Jun 29;9(1):2548. doi: 10.1038/s41467-018-04882-6. PubMed
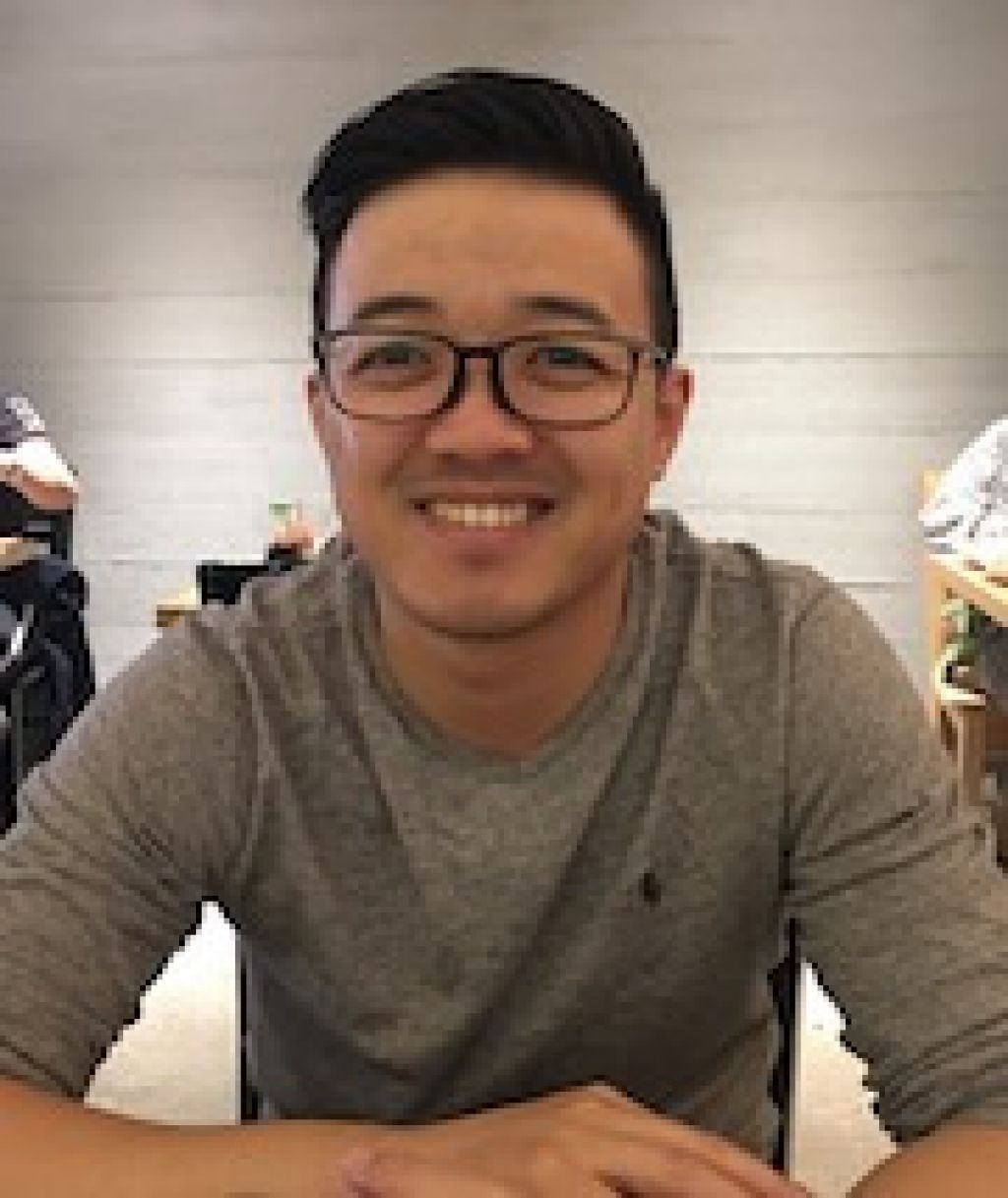
HiCRep: measuring the reproducibility of Hi-C genomic data
Summary by Tao Yang: Thanks to the soaring of genomic sequencing technologies, scientists nowadays have a great chance to demystify many aspects of the biology of genome. In recent years, a new high through-put sequencing technology named Hi-C has become increasingly popular, as it enables scientists to investigate interactions between almost any two loci of the genome. Hi-C promotes understanding of several mechanisms of the genome such as gene regulation, genome organization, and chromosome folding.
Behind the excitement, one should never forget the fundamental principle of science – reproducibility, that is, the data from two independent experiments of same conditions should be similar enough, if not identical. A solid biological conclusion should always be drawn from reproducible experiments. Then how do we evaluate the reproducibility of Hi-C data?
Over the years, correlation coefficients are widely used to evaluate the reproducibility of genomics data. At the early stage, correlation was also used to evaluate Hi-C data reproducibility. Quickly scientists realized that the correlation coefficient not a suitable measure. The special structure is induced by a distance-dependence effect – two loci with close proximity are more likely to have a high signal than that of far distance. Because of this phenomenon, two irrelevant Hi-C datasets can have a high correlation since they both are related to the common factor of distance.
To address this issue, Yang and colleagues designed a computational tool HiCRep that systematically accounts for this distance-dependent feature of Hi-C data. HiCRep first denoises the Hi-C matrix by smoothing, and then uses an innovative statistics named stratum-specific correlation coefficient (SCC) to quantify the similarity between two Hi-C datasets. To test the method, the research team evaluated the similarity of Hi-C data from several different cell types using HiCRep and the correlation statistics. Whereas the correlation statistics were bewildered by spurious correlations due to the distance-dependence effect, HiCRep was able to reliably differentiate the cell types. Additionally, HiCRep could accurately quantify the amount of difference between cell types and recapture the relative relationships between the cells. This research work is recently published on the journal Genome Research.
The leading author Tao Yang is a graduate student in Bioinformatics and Genomics program at Penn State. Yang is advised by Dr Qunhua Li and Dr Feng Yue. Other contributors include Feipeng Zhang, Fan Song, Ross C. Hardison at Penn State; and Galip Gürkan Yardimci and William Stafford Noble at the University of Washington. The research was supported by the U.S. National Institutes of Health, a Computation, Bioinformatics, and Statistics (CBIOS) training grant at Penn State, and the Huck Institutes of the Life Sciences at Penn State.
Yang T, Zhang F, Yardimci GG, Song F, Hardison RC, Noble WS, Yue F, Li Q. HiCRep: assessing the reproducibility of Hi-C data using a stratum- adjusted correlation coefficient. Genome Res. 2017 Aug 30. pii: gr.220640.117. doi:10.1101/gr.220640.117. [Epub ahead of print] PubMed
Other news article about this publication
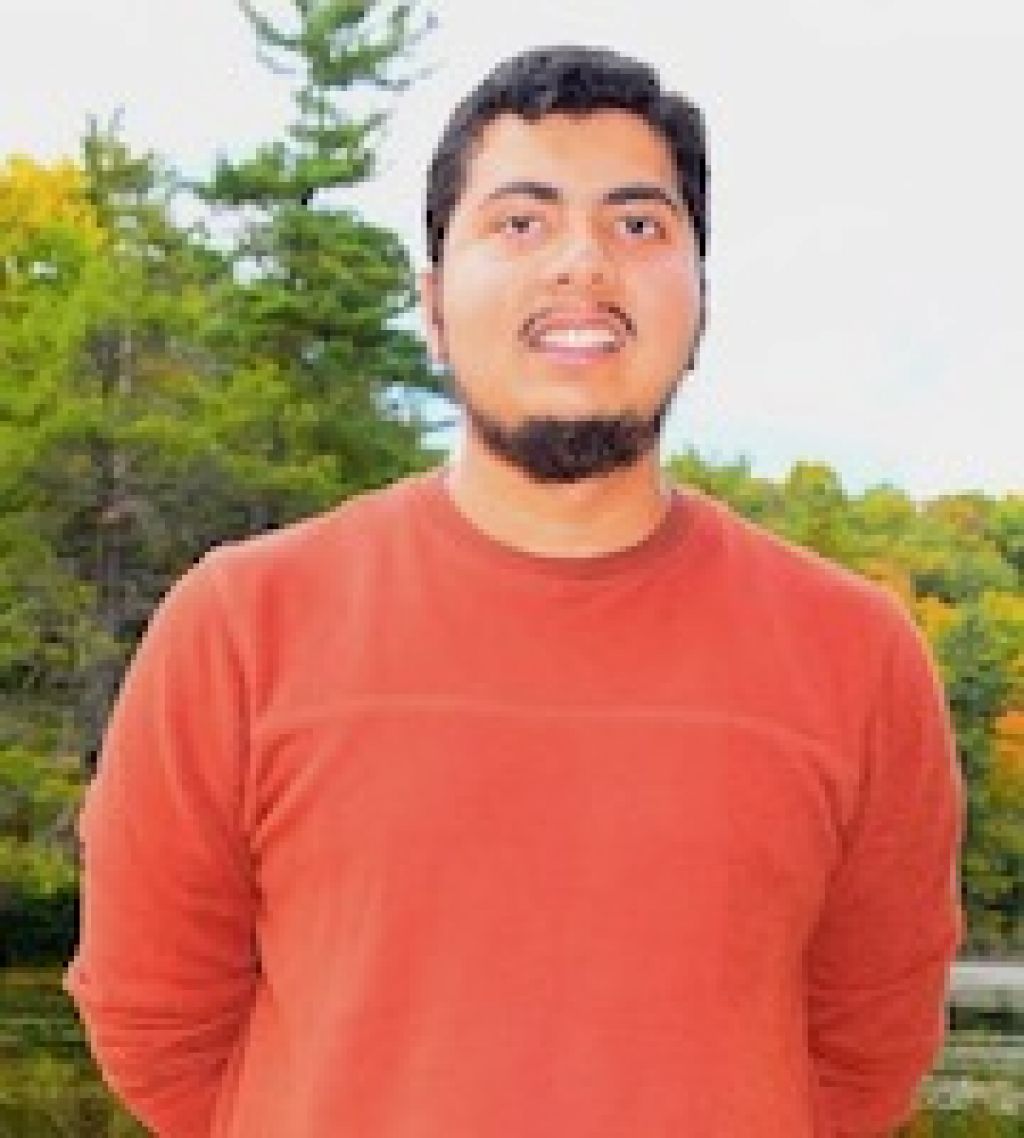
Evolutionarily encoded translation kinetics coordinate chaperone binding to nascent proteins
Summary by Nabeel Ahmed: Proteins play an integral role in functioning of a cell. They are synthesized on a ribosome where an mRNA sequence is translated to a protein. It has long been assumed that the structure and function of the protein is determined only by the sequence of the protein. We are trying to show that the speed at which protein is synthesized can also affect the folding of the protein into its right structure. Advances in Next-Generation Sequencing methods have allowed us to study the process of translation globally in the cell. Ribosome Profiling is the method which determines the location and number of active ribosomes translating a codon on all mRNAs at a particular time. My research is focused on developing methods to model this data and extract the rate of translation of every codon in the coding sequence of an mRNA. We can then investigate the molecular factors which influence the rate of translation and how they act together to result in the variability of translation rates along the mRNA. We also investigate the role of translation kinetics in co-translational processes like chaperone binding, protein folding and translocation. These findings can help us better understand the mechanism and regulation of protein synthesis in the cell.
This study has three major implications. Firstly, it highlights the broader role of the eukaryotic Hsp70 chaperone Ssb in folding of much larger number of newly synthesized proteins than previously expected. Secondly, it elucidates molecular principles of Ssb binding to the nascent polypeptide exiting from the ribosome's exit tunnel and its interdependence with other factors in the chaperone network. Finally the coordination between the translation kinetics and binding of Ssb reveals that the regulation of chaperone binding is encoded within the translation rate profile which is determined by mRNA sequence of a gene. The results from this study uncover several important molecular principles of chaperone binding to efficiently fold nascent polypeptides into fully functional protein structures.
This discovery suggests that the root cause of some diseases may be due to mutations that alter the speed of protein synthesis without changing the sequence of the protein (called synonymous mutations) thus possibly leading to inefficient binding of chaperones resulting in mis-folding of the protein structure. This opens up new avenues of biomedical research investigating new molecular mechanisms of disease.
In the field of synthetic biology, the molecular principles of Ssb chaperone binding to newly synthesized proteins can direct design of artificial chaperone scaffolds for guided folding of proteins in artificial systems.
Döring K, Ahmed N, Riemer T, Suresh HG, Vainshtein Y, Habich M, Riemer J,Mayer MP, O'Brien EP, Kramer G, Bukau B. Profiling Ssb-Nascent Chain Interactions Reveals Principles of Hsp70-Assisted Folding. Cell. 2017 Jul 13;170(2):298-311.e20. doi: 10.1016/j.cell.2017.06.038. PubMed